Back to Projects List
Multi-stage deep learning segmentation of teeth
Key Investigators
- Daniel Palkovics (Semmelweis Medical University)
- Csaba Pinter (Ebatinca)
- David Garcia Mato (Ebatinca)
- Andres Diaz-Pinto (NVidia)
Project Description
Segmenting and identifying the teeth in a mandible or maxilla is a difficult task, especially due to the high number of structures and their similarity. Recent results suggest that multi-stage segmentation may yield more accurate segmentation in these scenarios.
Objective
The idea is to create a simple two-stage approach in MONAILabel where the first stage detects the teeth centre and the second stage accurately segments the teeth themselves.
Approach and Plan
- Discuss with Andrés the details about multi-stage segmentation in MONAILabel
- Design the changes to be made
Progress and Next Steps
- Discussion with Andrés about multi-stage deep learning approach
- Multistage approach is more robust because the complexity is separated (robustness is the main advantage)
- Paper (see below) has several models: ROI, Centroid/Skeleton (numbers OR images), Multi-task tooth segmentation, Tooth ID classification, Cascaded bone segmentation
- Baseline data for centroid model are just the centroids, that can be calculated from the baseline segmentation. Same with the centerline one
- Implementing generic multi-stage approach in MONAILabel is a bit of a work
- In MONAI core this is easier to set up
- Why one model? Having one upper and one lower is OK for us
- Even lower + upper + bone + implant/tooth separation is a possibility
- Advantage of one multi-stage model is that we have only one system
- If we have both upper and lower then we need more data
- Clinically we’ll only have either upper or lower
- How do we connect the stages?
- Centroid/skeleton using numbers (not images) is regression (not segmentation)
- Concatenate input numbers on the “bottom of the UNet” where we have a huge array of numbers after downsampling
- MONAI files
- .pt: model (need to define network first etc.)
- .ts: torch script that contains preprocessing and the inference too
- Proposal
- Simple multi-stage model implementation using MONAI
- Initial: ROI definition -> Centroids -> Tooth segmentation
- Later still possible to add for example tooth identification, centerline, implant segmentation, etc.
Illustrations
There are some promising preliminary results
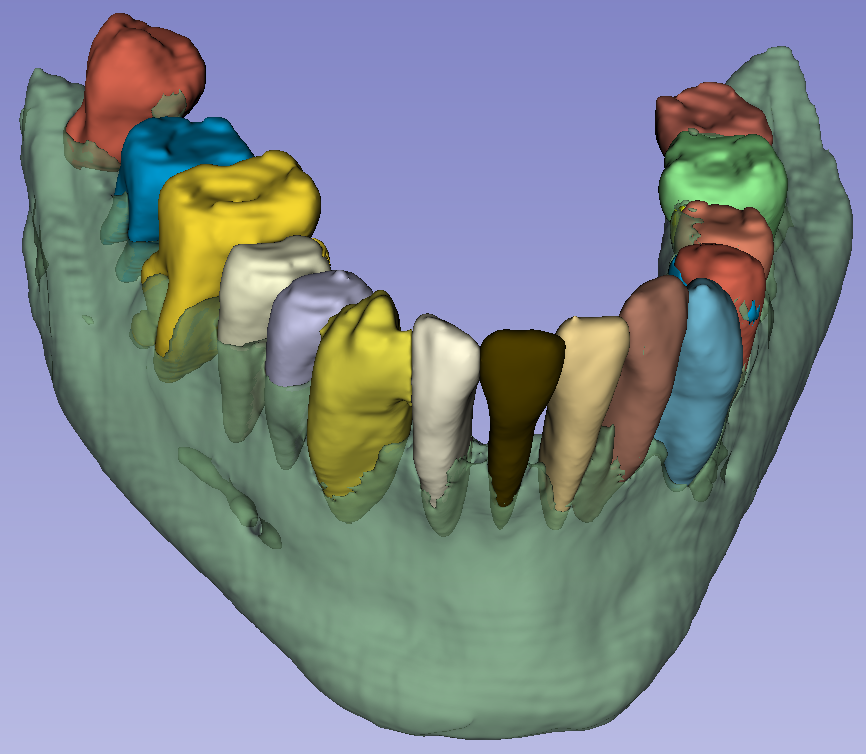
but there is room for improvement!
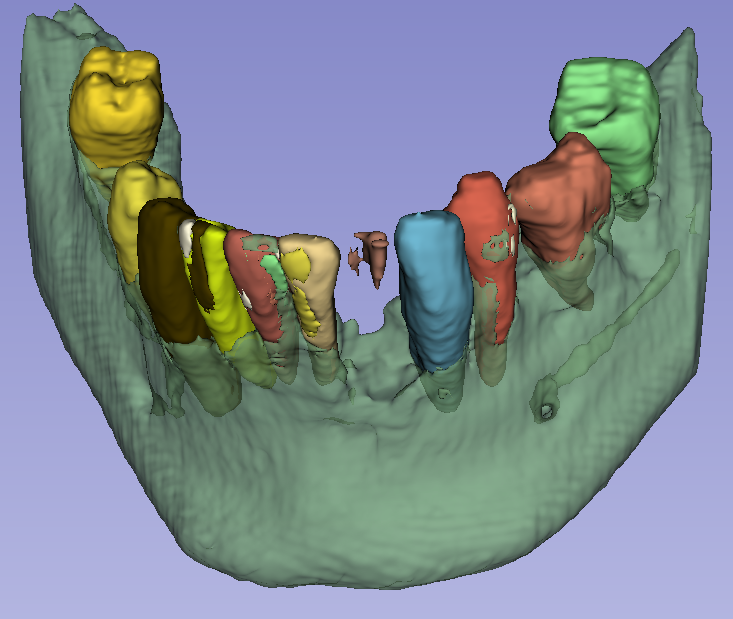
Background and References